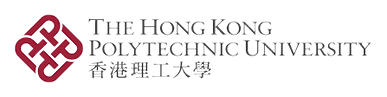
Yang's Group | Materials Informatics Lab
Research
High-throughput first-principles calculations accelerated functional materials development
Our group has been developing a python-based framework for high-throughput first-principles calculations (https://github.com/bitsoal/VASP_HTC_framework), and we have employed this semi-automatic framework to accelerate the innovation of various functional materials. For instance, we have screened 9 promising 2D metallic electrocatalysts for hydrogen evolution reaction (HER) from nearly 2000 2D materials.
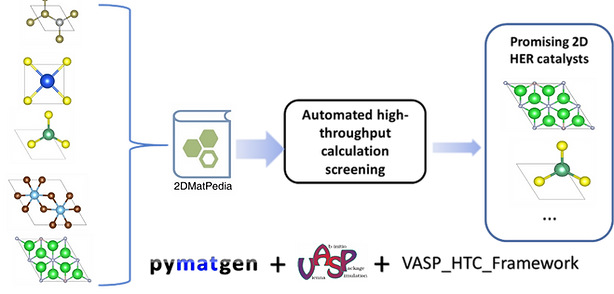
Our recent focus is on extending and combining high-throughput first-principles calculations with machine learning technologies, aiming to speed up development of catalysts towards a broad spectrum of (photo-)electrochemical reactions, such as HER, OER, ORR, CO2RR and NRR.
Ref.:
-
High-Throughput Identification of Exfoliable Two-Dimensional Materials with Active Basal Planes for Hydrogen Evolution, Tong Yang, Jun Zhou, Ting Ting Song, Lei Shen, Yuan Ping Feng* & Ming Yang*, ACS Energy Lett. 7, 2313-2321, 2020
-
High-Throughput Screening of Transition Metal Single Atom Catalyst Anchored on Molybdenum Disulfide for Nitrogen Fixation, Tong Yang, Ting Ting Song, Shijie Wang, Dongzhi Chi, Lei Shen*, Ming Yang*, & Yuan Ping Feng*, Nano Energy, 104304, 2020.
Synergizing DFT calculations and experiments to reveal underlying mechanism
Another research focus in our group is on revealing and understanding novel properties of emerging materials such as 2D materials and their heterostructures, perovskite oxide interfaces, and topological insulators by strongly interacting in-depth DFT calculations and intensive experiments. Our group is also engaged in developing high-throughput experimental growth methods for electrocatalysts and physical vapor deposition (PVD) for 2D monolayers on a large scale.
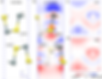
Ref.:
-
Gate-Tunable In-Plane Ferroelectricity in Few-Layer SnS, Yang Bao, Peng Song, Yanpeng Liu, Zhihui Chen, Menglong Zhu, Ibrahim Abdelwahab, Jie Su, Wei Fu, Xiao Chi, Wei Yu, Wei Liu, Xiaoxu Zhao, Qing-Hua Xu, Ming Yang*, & Kian Ping Loh*, Nano Lett. 19, 5109-5117, 2019.
-
Improving carrier mobility in two-dimensional semiconductors with rippled materials, Hong Kuan Ng, Ming Yang*, Jing Wu*, et al. Nature Electronics, 5, 489-496, 2022.
-
Deciphering the ultra-high plasticity in metal monochalcogenides, Lok Wing Wong, Ke Yang, Wei Han, Xiaodong Zheng, Hok Yin Wong, Chi Shing Tsang, Chun-Sing Lee, Shu Ping Lau, Thuc Hue Ly*, Ming Yang*, Jiong Zhao*, Nature Materials, 23, 196-204, 2024.
Physics-informed machine learning for functional materials innovation
Our research interest also includes the integration of physics and chemistry domain knowledge into the design of machine learning framework to improve the model's efficiency, interpretability, and generalizability. For example, recently, we have included the information of short-range interaction and passivation of dangling bonds into the graph representation, which results in much improved performance as illustrated in the below figure.
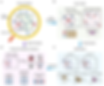